
Faculty
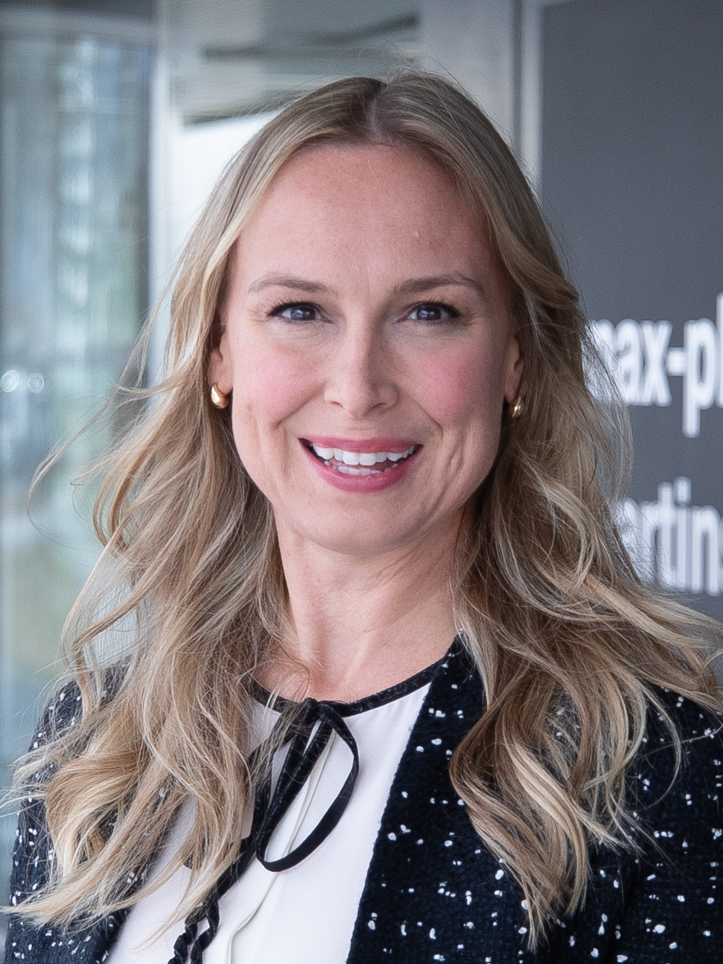
Jenna Wiens
Associate Professor
Computer Science and Engineering
University of Michigan, Ann Arbor
[email protected]
I am an Associate Professor of Computer Science and Engineering (CSE). I head the Machine Learning for Data-Driven Decisions (MLD3) research group. My primary research interests lie at the intersection of machine learning and healthcare. I received my Ph.D. in 2014 from MIT.
In 2015, I was named Forbes 30 under 30 in Science and Healthcare; I received an NSF CAREER Award in 2016, and in 2017 was named to the MIT Tech Review’s list of 35 Innovators Under 35.
PhD Students
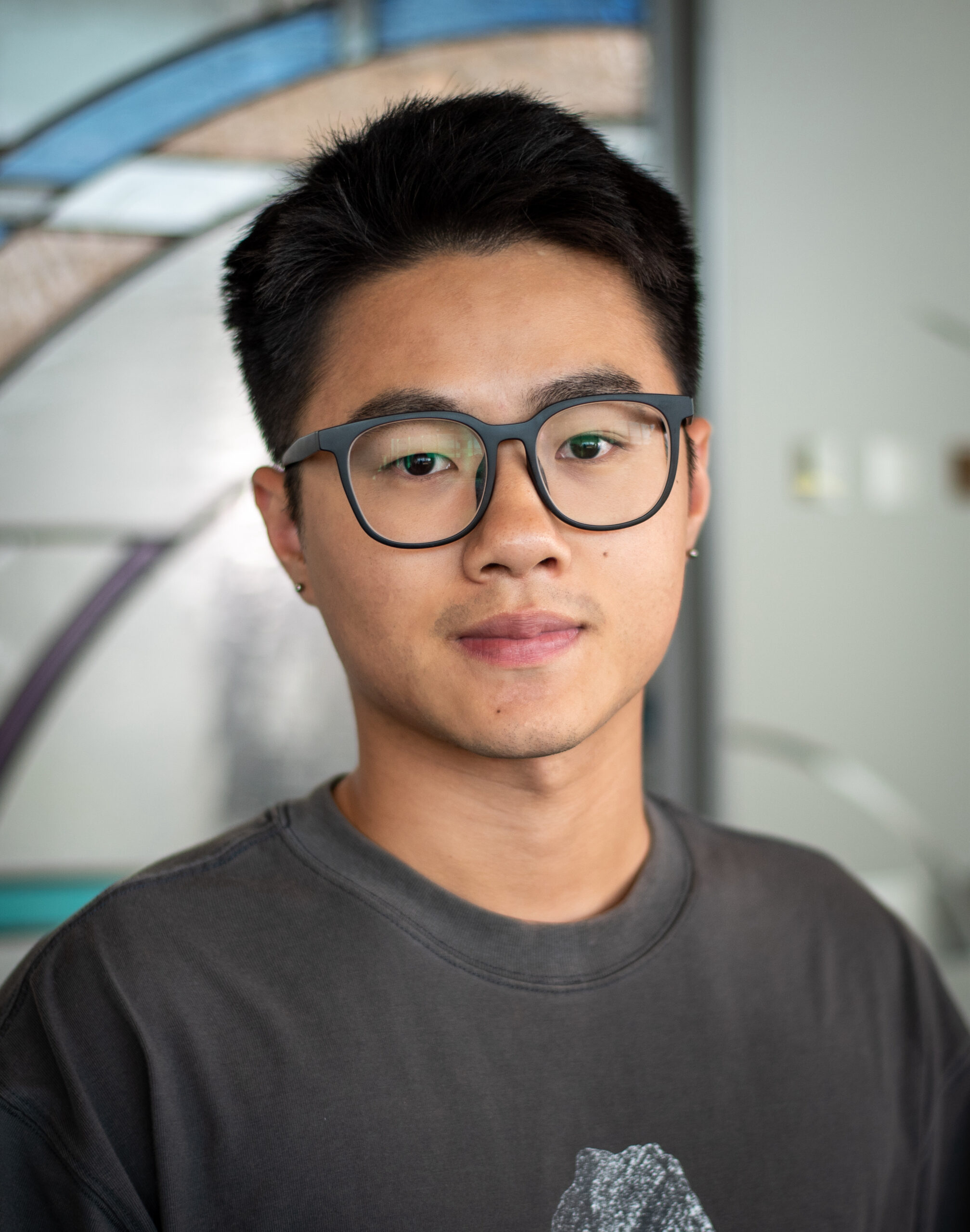
Donald Lin
Ph.D. Student
[email protected]
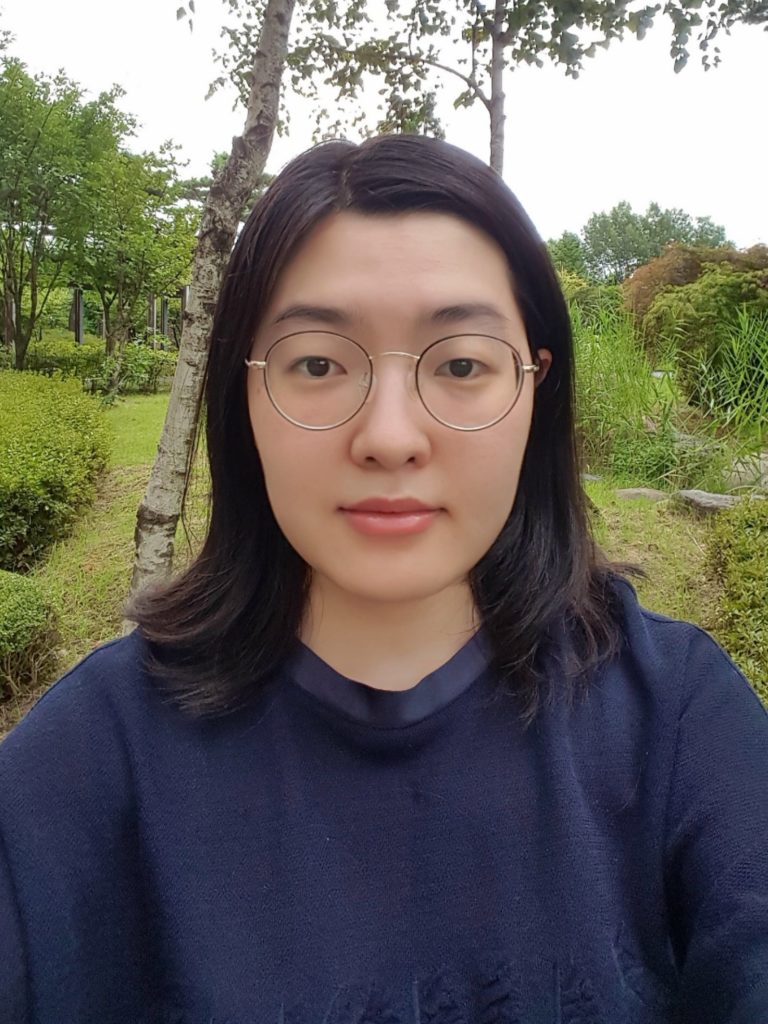
Jung Min Lee
Ph.D. Candidate
[email protected]
I’m a fifth-year Ph.D. Student in CSE. My research interest lies in applying machine learning techniques to healthcare problems to create actionable models clinicians can use. In the past, I’ve developed a classification model that uses brainwave signals to diagnose insomnia and identify cognitive impairment in patients.
Meera Krishnamoorthy
Ph.D. Candidate
[email protected]
I am a sixth-year Ph.D. candidate in CSE. My main research interest is using domain knowledge to inform the creation of novel machine learning methods. My current research project involves developing methods to represent genetic data. In particular, I am exploring the benefits of using machine learning approaches (over existing, non-machine learning approaches) that can leverage context to improve the utility of learned representations.
Michael Ito
Ph.D. Student
[email protected]
I am a third-year Ph.D. candiate in CSE. My research interests lie at the intersection of machine learning and healthcare. Currently, I’m working on graph representation learning and its applications in healthcare. In the past, I’ve worked on physics-informed machine learning, explainable artificial intelligence, and equivariant deep learning.
Sarah Jabbour
Ph.D. Candidate
[email protected]
Trenton Chang
Ph.D. Candidate
[email protected]
I am a fourth-year PhD candidate in CSE. My primary research interests are in machine learning robustness and fairness in the healthcare setting. In particular, I hope to incorporate socio-technical perspectives to design more equitable machine learning systems in this setting. In the past, I’ve worked on video machine learning robustness in the networking setting, neural text generation, and convex optimization for convolutional neural networks.
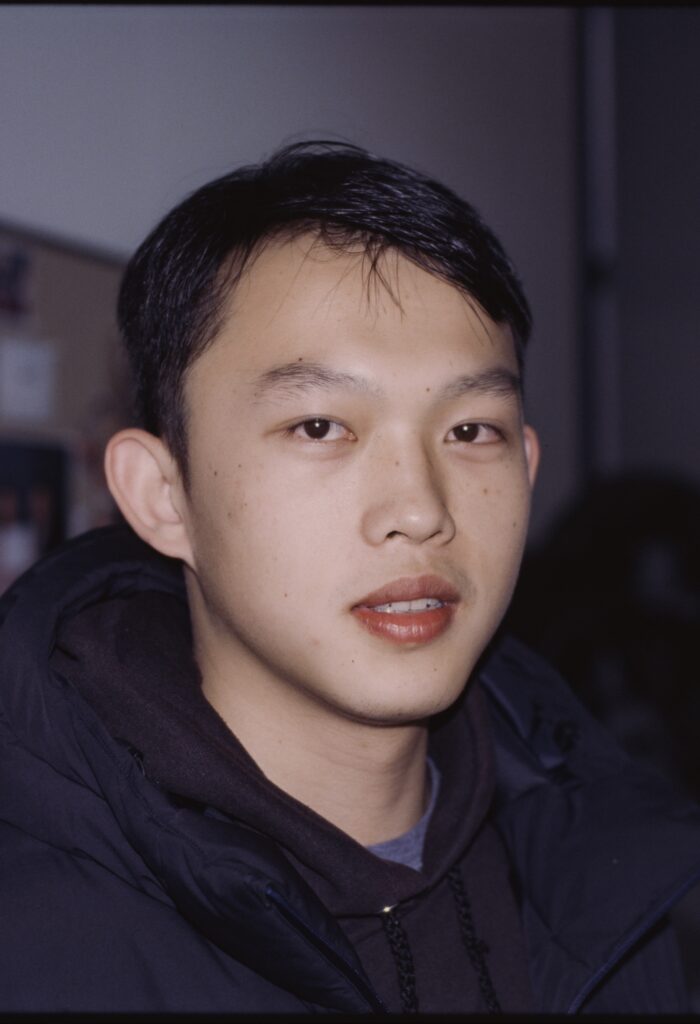
Winston Chen
Ph.D. Candidate
[email protected]
I’m a second-year Ph.D. candidate in CSE. My research interests are explainability and causality and their applications in healthcare. Currently, I’m building machine learning models to optimize hospital resource allocation and studying planning-based approaches for intervention decision-makings in social networks. Previously, I developed an error-controlled neural network interpretation method for biological hypothesis generation.
Research Specialists
Stephanie Shepard
Research Specialist
[email protected]
I am a Research Specialist in Computer Science and Engineering. In the MLD3 research group, I manage interdisciplinary projects with collaborators at Michigan Medicine and support the submission of grant proposals and research progress reports.
In 2017, I received my Ph.D. in Social-Personality Psychology from the University of Rochester. Using my expertise in human subjects research, I also support the MLD3 research group with predictive model evaluations that leverage methodologies such as vignette surveys and semi-structured interviews. I also contribute to qualitative research analyses.
Research Assistants
Gregory Kondas
Research Assistant
[email protected]
I am a full time research assistant in MLD3. My research focuses on machine learning with applications in healthcare. I am currently interested in foundation models for healthcare and their applications.
I earned my B.S. in Computer Science with a minor in Physics from the University of Michigan in 2024. (Go Blue!) In the past, my interest in science led me to a variety of research fields. I worked on problems in computational neuroscience with Professor Thad Polk. I also spent a summer optimizing the construction and testing of components for CERN’s upgraded muon spectrometer under the direction of Professor Bing Zhou.
Alumni

Donna Tjandra
Ph.D. [thesis]
Postdoctoral Fellow at the Veterans Affairs
[email protected]
I obtained my Ph.D. in CSE from U-M in June 2024. My main research interests include developing techniques to leverage electronic health record data and modeling disease progression in the context of survival analysis and noisy labels. During my PhD, I worked on projects that build on the literature from survival analysis for multiple events and noisy labels. This setting is important in clinical practice since accurate survival models can be used to identify those at risk of developing specific diseases well before symptom onset.
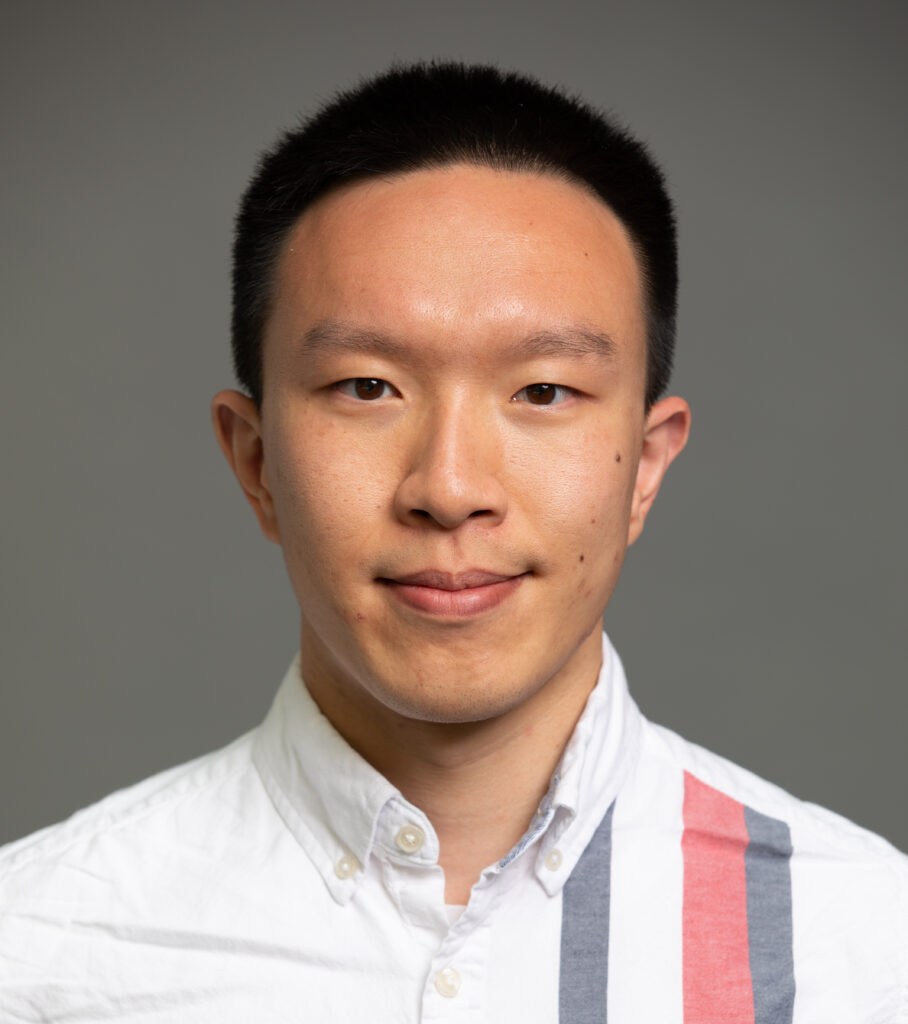
Shengpu Tang
Ph.D. [thesis]
Assistant Professor in the Department of Computer Science at Emory University
[email protected]
I obtained my Ph.D. in CSE in June 2024. My research focuses on developing computational methods for important problems in healthcare, including risk stratification of acute graft-vs-host disease, and dynamic treatment recommendations for acute dyspnea. Currently, I am investigating algorithmic solutions for a clinician-in-the-loop decision support system, by providing more choices to clinicians/patients that help improve patient outcome.
Harry Rubin-Falcone
Ph.D. [thesis]
[email protected]
I obtained my Ph.D. degree in CSE in November 2023. My research interests include time-series forecasting, machine learning for mental health, and diabetes research. I am currently working on forecasting blood glucose levels in type 1 Diabetes using deep neural networks, with the ultimate goal of facilitating improved blood-glucose management.
Fahad Kamran
Ph.D. [thesis]
Research Scientist at Apple
[email protected]
I obtained my Ph.D. degree in CSE in November 2023. My main research interests include adapting recent advancements in machine learning to build novel algorithms tailored to survival analysis and causal inference. Currently, I am developing approaches for the accurate estimation of individualized treatment effects to assist clinicians in matching individuals to treatments. In addition, on the more applied side, I explore the use of machine learning and data from wearable sensors to detect, predict, and prevent physiological harm.
Erkin Otles
MD-Ph.D. [thesis]
[email protected]
I am a Medical Scientist Training Program Fellow (MD-PhD student) at the University of Michigan. I have completed the first three years of my medical school training and obtained my Ph.D. in August 2022. My research interest lies in creating machine learning and artificial intelligence tools for patients, physicians, and health systems. Most of my work focuses on the development, deployment, and prospective validation of dynamic health outcome prediction models (e.g. early warning systems). I am co-advised by Jenna Wiens (Computer Science and Engineering) and Brian Denton (Industrial and Operations Engineering). I have a professional background in health IT development and hold a Master’s of Engineering from the University of Wisconsin.
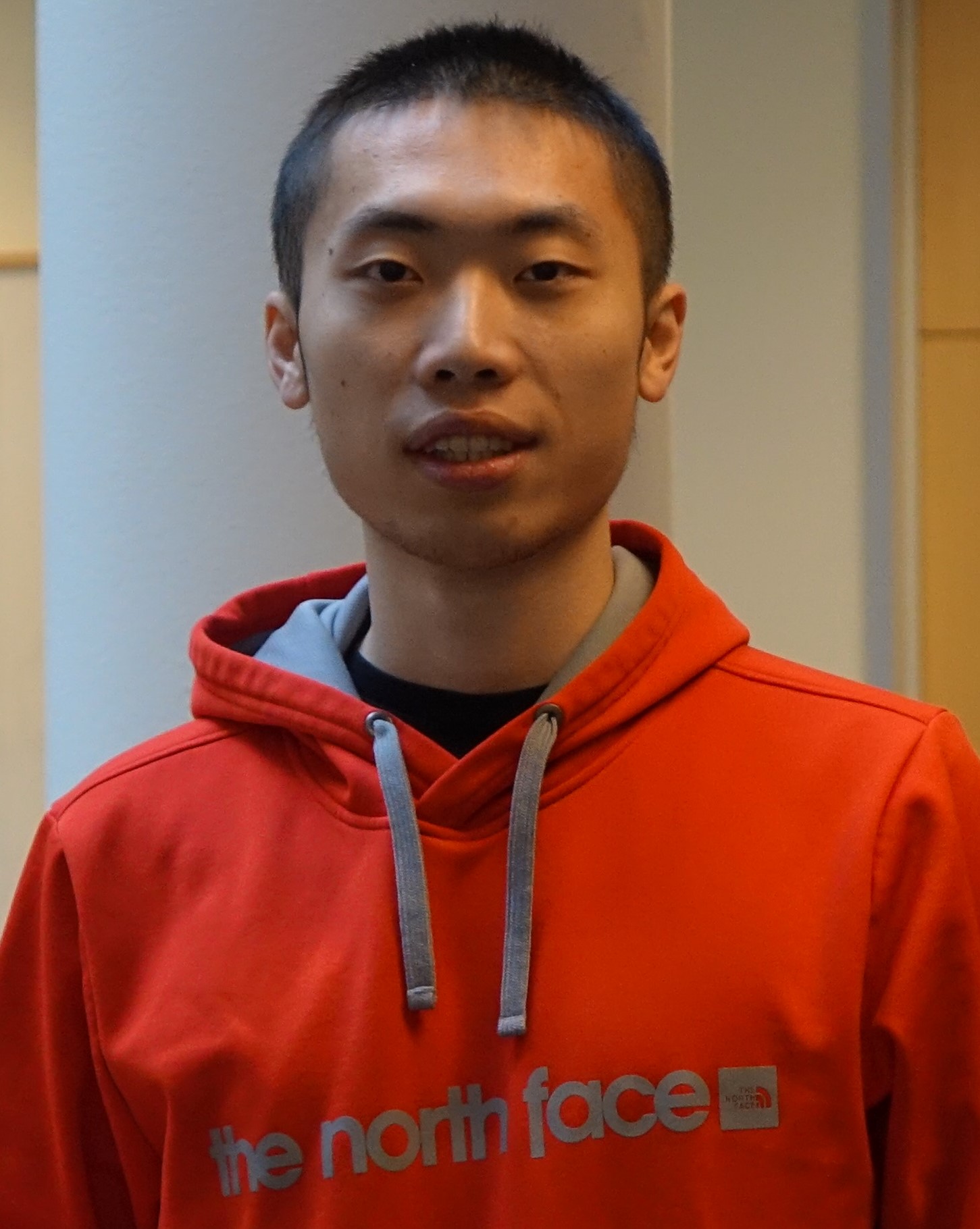
Jiaxuan Wang
Ph.D. [thesis]
Research Scientist at GE
[email protected]
I obtained my Ph.D. degree in CSE in February 2022. My main research interests include model interpretability, dataset shift, and non-convex optimization. Currently, I’m developing approaches to tackle covariate shift, which commonly arises in healthcare applications when the demographic composition changes across institutions. Models that can automatically adapt to this kind of shift offer more robustness and ultimately will require less maintenance once deployed.
Jeeheh Oh
Ph.D. [thesis]
Research Scientist at Paravision
[email protected]
I obtained my Ph.D. degree in CSE in February 2021. My thesis research focused on the development of novel machine learning algorithms for leveraging clinical time-series data. Clinical data is often limited and using domain knowledge to improve algorithms can benefit performance in these small sample settings. Specifically, I have developed novel architectures that can learn temporal invariances, time-series alignment, as well as to adapt to time-varying tasks. These methods have been used to predict adverse outcomes in clinical settings such as the risk of infection or mortality.
Ian Fox
Ph.D. [thesis]
Research Scientist
Facebook Applied AI Research
I obtained my Ph.D. degree in July 2020. I’m interested in ways to represent and control physiological signals, with a particular emphasis on blood glucose data collected from individuals with diabetes. As diabetes becomes more common throughout the world, such approaches are critical to helping improve outcomes and reduce patient burden. I have developed deep learning-based approaches to multi-step blood glucose forecasting, time-series representation techniques, and novel semi-adversarial approaches to classification. I have also done work using deep reinforcement learning to analyze NBA player tracking data. In my current project, I am working on developing and applying deep reinforcement learning methods to the problem of blood glucose control. My work has been published in KDD, IJCAI, and ICML.
Pets
Nemo Kondas, Dog
Hi! My name is Nemo. As in, “Finding Nemo”, except for imagine if you lost a dog instead of a fish. I am the newest lab member of MLD3, and I recently became roomies with Greg Kondas. I know my Marv (below) used to be the ONE and ONLY dog of this lab. Not anymore! Anyways, I’m still exploring my main research interests, but this whole genAI hype seems like a promising direction. I’ve been trying to use it to generate dog treats, but for some reason I can’t really figure out why they’re only 2D and I can’t bite into them through the computer screen.
Donnell Tang, Cat
Hi! My name is Donnell, a 4 year old cat living with my dad, Shengpu Tang. My main research interests lie at the intersection of machine learning and reinforcement learning, specifically in the context of how to use machine learning to optimally get the most treats from my dad. I know this research area is VERY exciting, so if you’d like to learn more, you can ask my dad about it. Unless you speak purr, then you can email me directly.
Taylor Ito, Cat
Hi! My name is Taylor, a 4 year old cat living with my dad, Michael Ito. My main research interests lie at the intersection of machine learning and napping on my dad’s keyboard. In my free time, I like to play with my cat toys and give my dad pep talks to help him through his PhD. In fact, I think he should dedicate his dissertation to me. You know what they say: anything is paw-sible with a cat by your side!
Marv Rubin-Falcone, Dog
Hi! I’m Marv, the ONE and ONLY dog of this lab. My dad is Harry Rubin-Falcone, and I’m his best bud. My main research interests lie at the intersection of machine learning and chewing on shoes. In my free time. I love playing music with my dad (who is a bassist), but for some reason he won’t let me play in gigs with him. Maybe one day I’ll form my own band, but for now, I’m going to spend my days sitting by my dad, reading his thesis.
MLD3 Throughout the Years


